General problem
A gesture has strong relationship not only with an actor’s motion, but also with the situation surrounding the actor. Traditionally, most gesture recognition methods focus on motion features only, and assign the gesture label based on the discriminative analysis. There are, however, many gestures which cannot always be uniquely determined by using motion features alone. For instance, considering gestures in a kitchen, "mixing something" and "sauteing something" would be done by similar motion sequences of a hand, which moves around in a circular motion. If the cook uses a bowl, the gesture label (we call it "cooking motion" in the contest) should be "mixing something in a bowl". Likewise, if he/she takes a frying pan, the label would be regarded as "sauteing something in a frying pan". Thus, the gesture label should be estimated by consideration of both the motion features and the context situation in which is it performed.
The contestants are expected to evaluate the human gestures in a kitchen from continuous video sequences. Five-types of cooking menus using the ingredients of egg, milk, and ham are considered in the contest. The candidates of the cooking menus are "ham and eggs", "omelet", "scrambled egg", "boiled egg" and "Kinshi-tamago". An actor cooks one of cooking menus with above ingredients. Each video contains several cooking motions: pouring, mixing, boiling, sauteing and so on. Using the testing videos, contestants have to establish the relationships between motion features and scene features (i.e. scene contexts) and achieve scene context based cooking motion recognition by assigning a correct cooking motion label to each video frame.
We are sure that scene context based gesture recognition can be applied for various uses: real-time analysis of a cooking scene or other types of scenes will enable such a system to advise a beginner what he/she should do at the next step including recovery of mistakes. Scene analysis and classification of recorded videos can also provide context-based segmentation of image sequences, and facilitate automated scene annotations for video databases. The context-based approach is applicable to other domains, for example; hospital operating rooms in medical practices, agricultural and manufacturing operations, etc.
Dataset
There are five candidate cooking menus. Each menu is cooked by five different actors; that is, five cooking scenes are available for each menu. The scene is captured by a Kinect sensor providng synchronized color and depth image sequences. Each of the videos are from 5 to10 minutes long containg 9,000 to 18,000 frames. A cooking motion label is assigned to each frame by the organizers, indicating the type of gesture performed by the actors. These labels are regarded as ground truth in the contest.
Competition tasks
Contestants must evaluate the testing video sequence in order to estimate the cooking motion in each frame. Of course, scene contexts including ingredients, cooking tools and motions should be extracted from the training video sequences in advance. Using the scene context is not an indispensable condition, but the organizers strongly recommend utilizing it. The following figure shows a brief example of cooking an “omelet”. The task of the contest is to recognize the “cooking motion label” in the middle layer of cooking hierarchy.
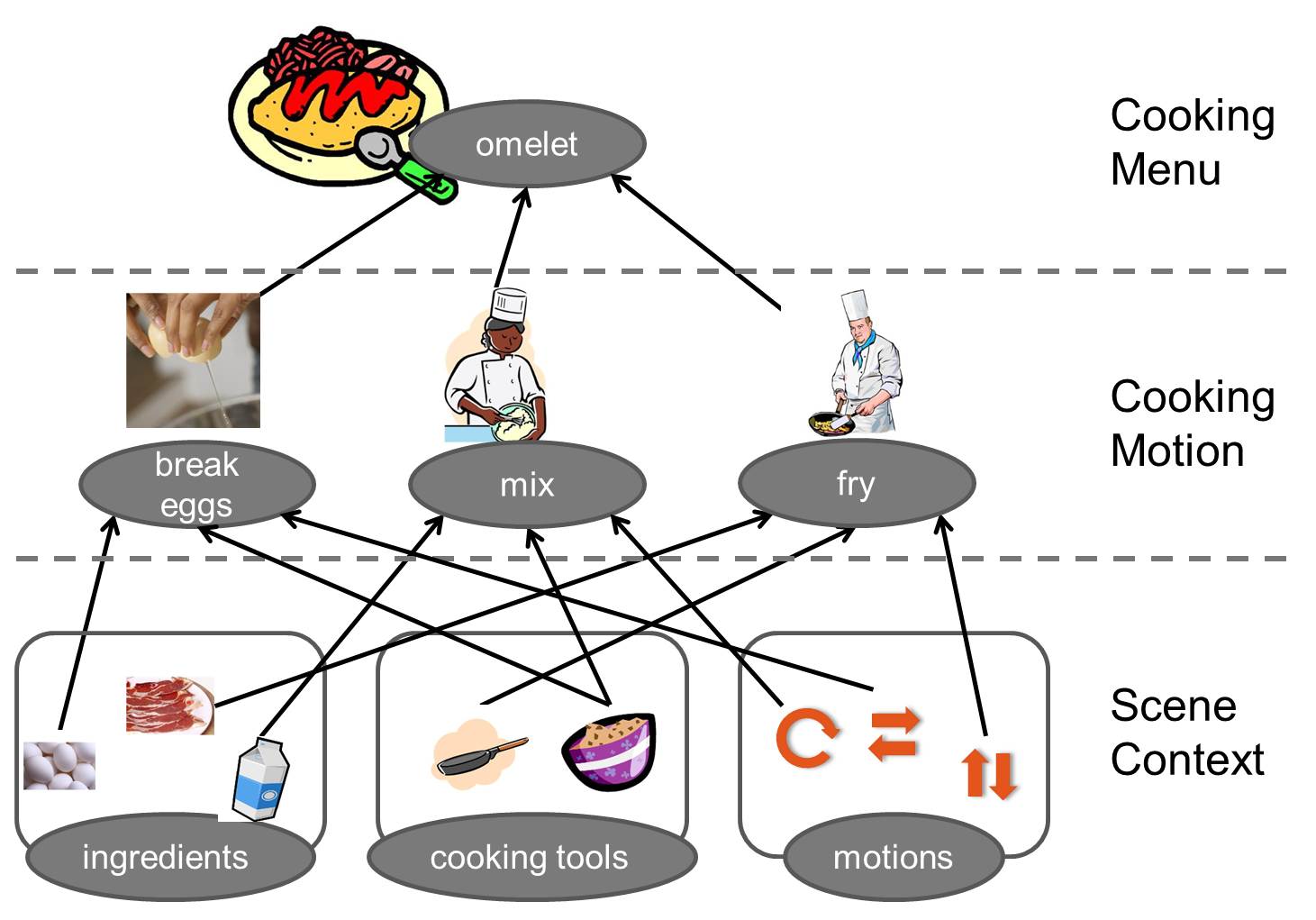
To submit the results, contestants have to follow the instructions described in the participation page.